
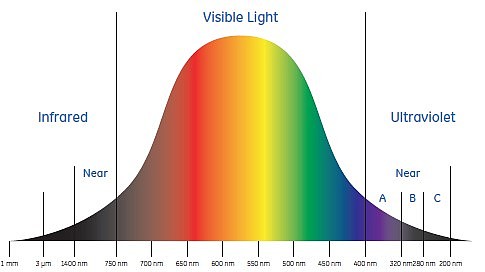
- SEQUENTIAL TESTING MULTINOMIAL DISTRIBUTIUON HOW TO
- SEQUENTIAL TESTING MULTINOMIAL DISTRIBUTIUON FREE
Often in a high dimensional feature set, there remain several features which are redundant meaning these features are nothing but extensions of the other essential features. Now, with this high dimensionality, comes a lot of problems such as - this high dimensionality will significantly increase the training time of your machine learning model, it can make your model very complicated which in turn may lead to Overfitting. This type of dataset is often referred to as a high dimensional dataset. The importance of feature selection can best be recognized when you are dealing with a dataset that contains a vast number of features. Understanding the Importance of Feature Selection Implementation of different feature selection methods with scikit-learnįeature selection is also known as Variable selection or Attribute selection.Įssentially, it is the process of selecting the most important/relevant.Different types of feature selection methods.Difference between feature selection and dimensionality reduction.Introduction to feature selection and understanding its importance.You got an informal introduction to Feature Selection and its importance in the world of Data Science and Machine Learning. In many of the cases, Feature Selection can enhance the performance of a machine learning model as well. So, what's the solution here? The most economical solution is Feature Selection.įeature Selection is the process of selecting out the most significant features from a given dataset. The machine model takes more time to get trained.These features act as a noise for which the machine learning model can perform terribly poorly.

Unnecessary resource allocation for these features.These features cause a number of problems which in turn prevents the process of efficient predictive modeling. It has been seen that the contribution of these types of features is often less towards predictive modeling as compared to the critical features. Often, in a high dimensional dataset, there remain some entirely irrelevant, insignificant and unimportant features.
SEQUENTIAL TESTING MULTINOMIAL DISTRIBUTIUON HOW TO
So, you might wonder with a commodity computer in hand how to process these type of datasets without beating the bush. But that is not the point of discussion here. You will find datasets where the number of features is very much, but they do not contain that many instances. The more the number of features the larger the datasets will be. So, what makes these datasets this large? Well, it's features. It becomes very challenging to process the datasets which are very large, at least significant enough to cause a processing bottleneck. Sometimes they are small, but often at times, they are tremendously large in size.
SEQUENTIAL TESTING MULTINOMIAL DISTRIBUTIUON FREE
If you want to learn more in Python, take DataCamp's free Intro to Python for Data Science course.
